Understanding Discovery Data Pricing: Strategies and Implications
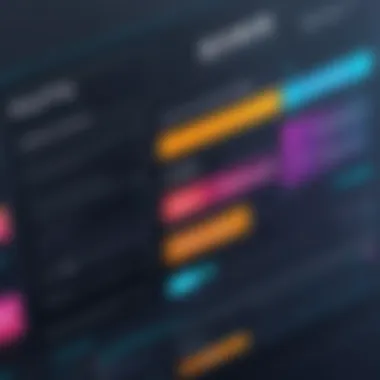
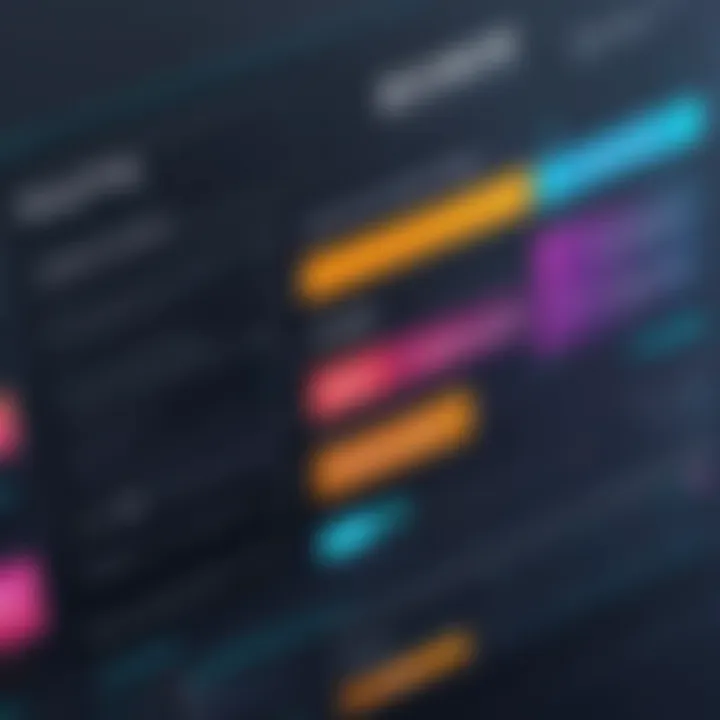
Intro
In today's data-driven economy, the way organizations price discovery data has far-reaching implications. Businesses, from a small startup to a global enterprise, must navigate this intricate landscape effectively. With the ever-growing demand for insights and analytics, understanding the mechanisms behind discovery data pricing is crucial. Not only does it affect budget allocation, but it also influences strategic decision-making processes across various sectors.
Discovery data pricing integrates a variety of factors, from the quality of data to the methodologies used in its valuation. Such pricing can often seem like searching for a needle in a haystack. Organizations need to grasp how these elements play into the costs associated with data acquisition.
This section introduces the key components of discovery data pricing, underlining the importance of factors that affect pricing strategies. Understanding these elements equips businesses with the insight needed to make informed strategic choices.
Software Overview
Despite the focus on data, it's critical to explore the software tools that facilitate data acquisition and analysis. Knowing how these tools function provides context to the pricing mechanisms that will be discussed later.
Software Features
Software designed for managing discovery data typically comes with features such as:
- Data Integration: Multiple sources are seamlessly connected, ensuring that data streams from various platforms merge efficiently.
- Real-time Analytics: This enables businesses to make timely decisions based on the latest insights pulled from the data sets.
- User-friendly Interfaces: Intuitive designs allow professionals, regardless of their tech-savvy backgrounds, to navigate the tool effectively.
Understanding these features is key to identifying why certain tools might carry a higher price tag than others. For instance, a package offering real-time data insights could command a premium compared to one that simply aggregates datasets.
Technical Specifications
When evaluating software options, certain technical specifications can serve as benchmarks. These include:
- Data Capacity: Ability to process large volumes of data efficiently.
- Cloud Compatibility: An essential feature today, as it allows for flexbility in usage and storage.
- Security Protocols: Robust measures must be in place, ensuring data privacy and protection against breaches.
By assessing these technical specs, businesses can better evaluate the total cost of ownership, leading to a more comprehensive understanding of pricing structures.
Peer Insights
To further enrich this analysis, it's helpful to look into peer experiences with discovery data tools. These insights offer practical examples that illustrate the implications of pricing strategies.
User Experiences
Experiences may vary widely based on the industry. Professionals in finance, for instance, often report higher satisfaction levels when using tools that provide deep analytics and forecasting capabilities. In contrast, businesses in sectors like retail might lean towards those that excel in real-time consumer behavior insights.
Pros and Cons
Every tool comes with its upsides and downsides, and being aware of these can be crucial for making informed choices:
Pros:
- Informed Decision Making: Better data equates to more reliable strategies.
- Competitive Advantage: Organizations leveraging advanced tools can stay ahead of competitors unexpectedly.
Cons:
- High Costs: Premium tools carry high upfront and ongoing subscription fees.
- Complexity: Some tools can become overwhelming, requiring dedicated training.
Understanding user insights adds depth to any analysis of discovery data pricing. It helps illuminate the trade-offs organizations must consider as they chart their data management strategies.
Prelims to Discovery Data
In the digital age, data has emerged as a cornerstone of informed decision-making for businesses, both big and small. Fast-paced advancements in technology mean that the realm of discovery data is increasingly relevant, even critical, to how organizations operate. This section draws attention to the fundamental aspects of discovery data, emphasizing its multifaceted nature and the considerable implications it holds for various sectors.
Defining Discovery Data
Discovery data can essentially be viewed as a treasure trove of information that organizations sift through to gain insights into customer behavior, market trends, or operational efficiencies. This term encompasses various data types collected through diverse means including surveys, online interactions, transactions, and social media activity. Companies leverage this data not only to understand what their customers want but also to make predictive analyses that can significantly shape their business models.
For example, consider a retail firm analyzing customer foot traffic data during different times of the day. It uses discovery data to determine peak hours, adjust staffing levels, and subsequently enhance the shopping experience. It’s not just numbers; it’s knowledge that can drive growth.
The Importance of Discovery Data
Understanding the role of discovery data cannot be overstated. Its importance lies in the actionable insights it provides that help organizations tailor their strategies to fit market needs effectively. Here’s where the interplay between data quality and decision-making becomes paramount. Organizations that fail to appreciate this may find themselves chasing shadows—making decisions based on incomplete or inaccurate data.
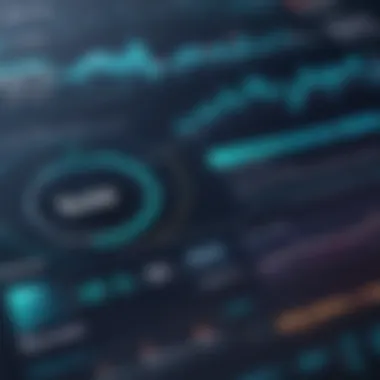
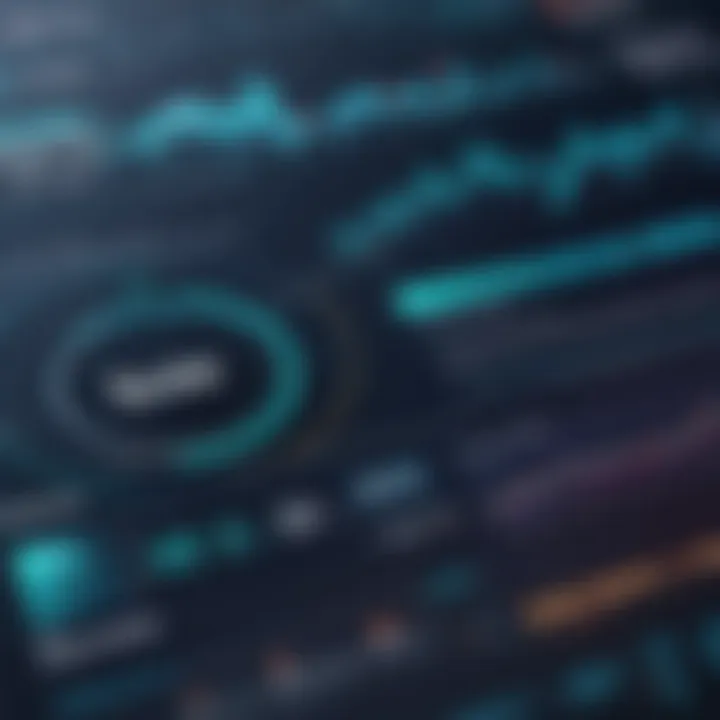
Moreover, the strategic implications can be far-reaching. With solid discovery data, organizations can:
- Enhance Customer Engagement: Tailoring products and services based on actual customer preferences rather than assumptions fosters stronger relationships.
- Streamline Operational Processes: Insight-driven adjustments can lead to efficiencies in resource allocation, cost management, and product development.
- Anticipate Market Shifts: Organizations able to recognize patterns in discovery data may spot trends before they become mainstream, offering a crucial competitive edge.
"In the world of data, those who can harness the insights effectively will lead the pack into the future."
In summary, discovery data serves as both a compass and a guide revealing uncharted territories in a business’s operational landscape. By skillfully navigating the information provided, companies set the stage for both operational excellence and sustained growth.
Understanding Data Pricing Models
Understanding data pricing models is crucial for organizations looking to harness the power of discovery data effectively. It's more than just numbers; it’s about determining value and carving out a strategy that aligns with business objectives. Pricing models not only dictate the cost but also shape perceptions regarding the value of the data your organization interacts with.
Getting the pricing model right can have significant implications. The right approach ensures profitability, promotes competitive edge, and helps stakeholders comprehend the real worth of data to their strategic initiatives. Each model has its strengths and weaknesses, which can deeply influence market positioning and customer relationships.
Cost-Plus Pricing
Cost-plus pricing is straightforward yet effective. This model involves calculating the total costs incurred during the data acquisition or generation process and then adding a mark-up to define the final price.
Let's say a company invests $10,000 in data acquisition, and they apply a 20% markup. This method guarantees that they cover their costs while ensuring profitability, ultimately arriving at a selling price of $12,000. It’s commendable for its simplicity but has limitations. This strategy lacks insight into market dynamics. If competitors are charging significantly less, a business may still overshoot their pricing without realizing it.
"Cost-plus pricing may protect you from loss, but it won’t shield you from competition."
Moreover, businesses using this model might overlook the intrinsic value the data holds for consumers. It provides a safety net but can lead to missed opportunities for higher returns.
Value-Based Pricing
Value-based pricing flips the script. Instead of focusing solely on cost, this model centers on the perceived value of data to the customer. Here, businesses need to engage in thorough market research and understand the end-user's needs and expectations.
For instance, if a company recognizes that their dataset enables a client to save $100,000 a year, they might set a price based on that potential value rather than a simple cost calculation.
By aligning the price with perceived value, businesses can capture more consumer surplus, often resulting in enhanced revenue. This method promotes deeper engagement with customers, fostering long-term relationships based on understanding and trust.
However, value-based pricing can demand constant market analysis to adapt to changes and ensure the pricing remains relevant. It requires a detailed grasp of customer motivations, making it a bit of a balancing act.
Competitive Pricing Strategies
Competitive pricing strategies involve setting prices based on what competitors charge for similar data offerings. Companies might research their competitive landscape thoroughly, aligning their pricing structure with those of rivals to capture or retain market share.
This strategy demands constant vigilance. Imagine a scenario where a competitor drops their prices. If a company doesn't adjust their pricing, they risk losing clients drawn to more affordable options.
While it helps organizations stay relevant in the market, relying solely on competitive pricing can be precarious. It could lead to price wars, squeezing margins thinner and minimizing profit. A business may eventually prioritize pricing over value, neglecting the quality or uniqueness of their data.
In summary, a comprehensive understanding of data pricing models empowers organizations to approach the market strategically. By evaluating different methodologies in the context of their own business needs and competition, they can optimize their pricing strategies for discovery data and drive success in an increasingly data-driven world.
Factors Influencing Discovery Data Pricing
Understanding the elements that sway the pricing of discovery data is crucial for any business, whether big or small, dabbling in data consumption. These factors are not just numbers on a spreadsheet but intricate links that connect quality, demand, and regulations. Let’s break this down, layer by layer, to reveal how each component holistically shapes discovery data pricing.
Data Quality and Accuracy
The old adage "you get what you pay for" rings particularly true in the realm of discovery data. The paramount determinant of pricing is the quality and accuracy of the data. When a dataset is pristine, with verified, up-to-date, and relevant information, businesses are often willing to pay top dollar for it.
Here’s the kicker: pricing also correlates with the costs incurred to maintain this high standard of data. For instance, top-tier firms may invest heavily in rigorous data cleaning and validation processes. That enables them to offer insights that are not just actionable but also reliable.
- High Accuracy: Leads to informed decision-making.
- Low Error Rates: Reduces the resources needed for correction and risk management.
Companies focusing on quality assurance often earn a premium, as clients recognize that poor data quality can lead to misguided strategies or, worse, costly mistakes. The intricacy lies in balancing the costs of maintaining quality while still being competitive in their pricing against peers who might offer lower-cost options without the same integrity.
Market Demand and Supply Dynamics
Supply and demand—it’s the backbone of economic principles, and yes, it extends its tendrils into the discovery data sphere. When market demand spikes—say, due to a sudden emergence of a new tech trend—the pricing for related data sees rapid escalation. Conversely, if numerous providers flood the market with similar data offerings, prices may plummet.
Factors to consider in this dynamic include:
- Sector Trends: Industries like healthcare might see a surge when new regulations are introduced, causing greater demand for compliant data.
- Economic Cycles: In a thriving economy, businesses invest more in analytics, thus pushing demand and prices higher.
- Technological Advancements: New platforms may usher in innovative ways to gather or interpret data, adjusting the market's equilibrium.
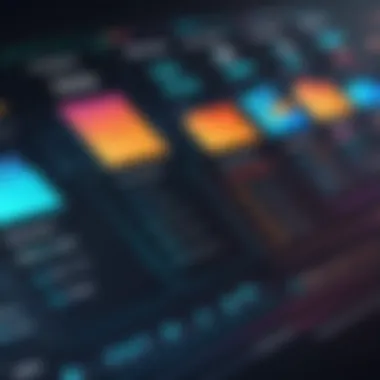
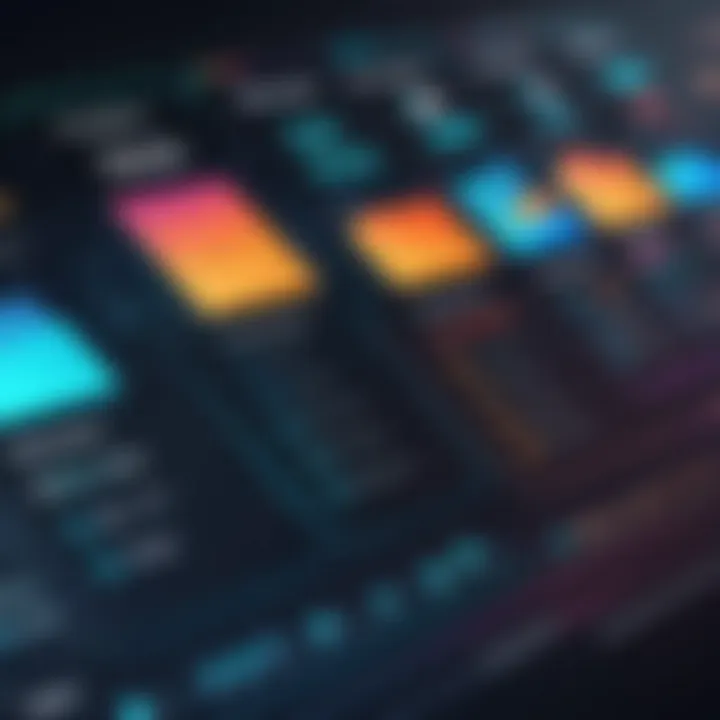
As competition ramps up, savvy providers must adapt. They might offer niche data sets or incorporate specialized analytics capabilities to maintain profit margins, touching upon both innovation and the art of pricing tactics.
Regulatory Considerations
In today’s data-centric world, you can’t overlook the heavy boots of regulation. Laws and standards, such as GDPR and CCPA, impose restrictions that can impact how data is collected, stored, and priced. These regulatory frameworks often necessitate additional measures, increasing operational costs, which ultimately reflect in the pricing strategies.
Considerations include:
- Compliance Costs: Expenses associated with conforming to legal mandates.
- Liabilities: Companies face penalties for breaches that can be severe, thus prompting higher data prices to cover risks.
- Transparency: Customers are becoming increasingly aware of their data rights, prompting providers to ensure they demonstrate compliance, influencing pricing structures further.
Regulatory pressures not only shape the costs associated with data offerings but can also affect consumer demand. As such, businesses navigating this landscape must maintain a keen awareness of regulatory shifts and how these developments reflect in their pricing strategies—and ultimately, their bottom lines.
"Knowledge of the laws governing data usage is as valuable as the data itself. No business can afford to ignore compliance in its pricing strategy."
The Role of Technology in Data Pricing
In the modern landscape of data valuation, technology is a pivotal player, shaping how businesses determine the worth of discovery data. The advent of sophisticated tools and methodologies aids organizations in not just managing costs but also in strategizing around their data usage. Emphasizing the role of technology serves as a cornerstone in understanding the intricacies of pricing mechanisms. This section will uncover methods that technology brings to the table, focusing on its significance in automating processes and applying data analytics effectively.
Automation and Data Analytics
Automation in data pricing allows organizations to streamline their pricing strategies. By implementing automated systems, businesses can avoid the tedious and often error-prone manual calculations. Automated tools simplify the process of monitoring market conditions and adjusting data prices accordingly. This leads to quicker responses in a fast-paced marketplace.
Benefits of Automation:
- Efficiency: Reduction in time taken for data pricing decisions can make all the difference. Automation brings precision.
- Consistency: Ensures that pricing models remain stable across time, preventing any potential inconsistencies from human error.
- Scalability: As businesses grow, automated pricing systems can adapt without significant extra cost or effort.
Consider the way a financial institution can update interest rates based on real-time data feeds using automated systems. By having the ability to respond instantly to market fluctuations, they can align their offerings with current demand levels, a move that is critical in maintaining competitive positioning.
Data analytics, on the other hand, enables firms to derive insights from the data they collect. Implementing robust analytics solutions allows companies to evaluate pricing models based on historical data trends, consumer behavior, and other pertinent metrics. The synergistic effect of automation and analytics contributes to an agile pricing strategy that reflects true market value rather than guesswork.
"The combination of data analytics and automation not only enhances accuracy but also cultivates a responsive pricing environment."
Artificial Intelligence Applications
Artificial Intelligence (AI) has emerged as a game changer in the realm of data pricing. Through predictive analytics, AI algorithms can analyze vast amounts of data to forecast pricing trends and consumer preferences. It is like having a crystal ball that provides businesses insights into what the market might look like tomorrow or next week.
Key Applications of AI in Data Pricing:
- Dynamic Pricing: AI enables real-time adjustments to pricing based on customer behavior, inventory levels, and competitor pricing. An online retailer might increase prices during peak demand but offer discounts when inventory is stagnant.
- Consumer Insights: Understanding buyer tendencies through AI means businesses can tailor their pricing strategies according to specific market segments. This personalization can lead to increased sales and improved customer loyalty.
- Risk Assessment: AI can assess market risks and help businesses create contingency pricing strategies that allow them to minimize potential losses.
Organizations are now running experiments using AI models to identify optimal price points—demonstrating the technology's value well beyond traditional pricing strategies. Through continuous learning from historical data, these AI systems can develop sophisticated pricing models that adapt as the marketplace evolves.
In summary, technology not only simplifies pricing approaches but elevates the entire concept of data pricing into a more analytical and responsive business function. The implications here are vast, affecting budgeting, decision-making, and strategic deployment of resources—all crucial for sustained business success.
Implications for Businesses
Understanding the implications of discovery data pricing is crucial for businesses aiming to leverage data effectively. This section dives into how pricing strategies affect budgeting, financial forecasting, and strategic planning. It helps organizations align their data initiatives with overall business goals while navigating the complexities of the data-driven marketplace.
Budgeting and Financial Forecasting
When it comes to budgeting and financial forecasting, businesses often grapple with forecasting data-related expenses. The cost of acquiring discovery data can swing widely based on several factors such as quality, accuracy, and availability. Poorly estimated data costs can lead to misallocated resources, which impacts overall financial health.
To mitigate these risks, organizations must adopt a proactive approach. For instance:
- Conducting Regular Assessments: Frequent evaluations of data requirements and costs allow businesses to adjust their budgets more accurately. Knowing what data is essential and at what cost helps in making informed financial decisions.
- Leveraging Data Analytics Tools: Tools like Tableau or Microsoft Power BI can offer insights into spending trends regarding data subscriptions or purchases. Companies can identify which data sources provide the best return on investment.
- Building Contingency Plans: Since data pricing can be unpredictable, businesses should set aside resources specifically for data purchases that may arise unexpectedly.
Investing time in these strategies will provide a clearer financial picture, keeping businesses flexible in their budgeting.
Strategic Decision-Making
The interplay between data pricing and strategic decision-making cannot be overstated. Companies that understand how their data costs impact their decision-making processes tend to be more innovative and agile. Businesses must consider the following:
- Alignment with Business Objectives: It's imperative to ensure data acquisition aligns with broader business strategies. Data should serve to drive decisions that enhance customer satisfaction or operational efficiencies.
- Influencing Product Development: Quality discovery data can lead to informed product development strategies. If a business understands customer preferences through data, they can create offerings that directly target consumer needs.
- Enhancing Competitive advantage: Organizations that effectively utilize high-quality data can outpace their competitors. It’s not just about having the data but understanding its value and integrating it into decision-making processes to stay ahead.
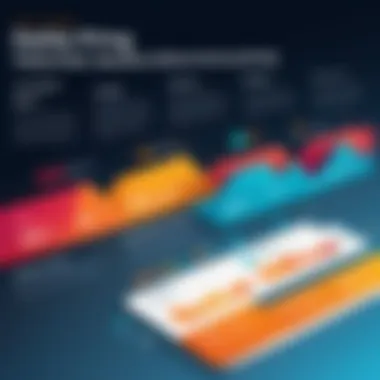
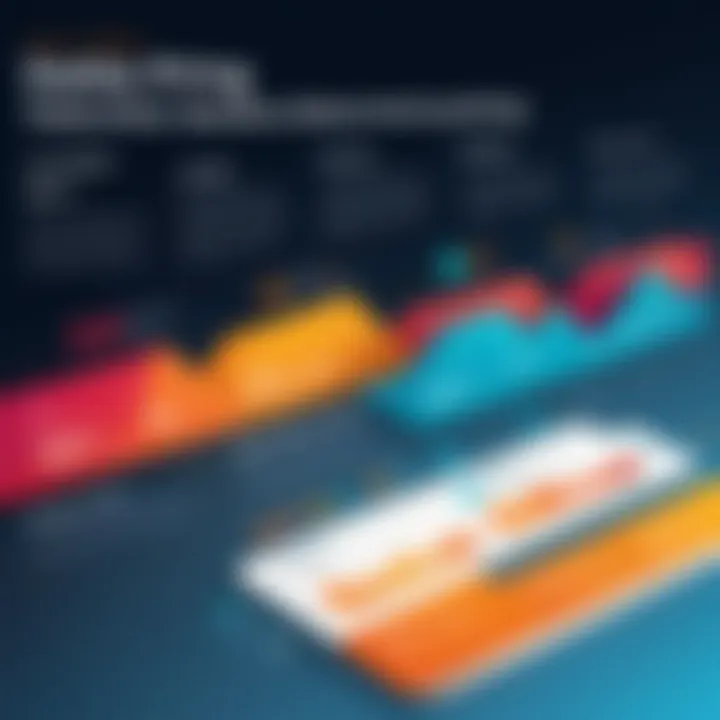
Effective data pricing strategies turn data from a cost center into a strategic asset.
Challenges in Discovery Data Pricing
Among the labyrinth of discovery data pricing, there arise various challenges that can make even the most astute professionals raise an eyebrow. These challenges, while daunting, are crucial to comprehend as they pave the way for better strategizing and operational management. Understanding these hurdles can empower both businesses and consumers to make informed decisions regarding data valuation and usage.
Data Privacy and Security Issues
In today's digitized world, data privacy and security are not merely afterthoughts; they are core concerns that demand attention. With the increase in cyber threats and violations, businesses operating in this space must tread carefully. Regulatory frameworks such as the General Data Protection Regulation (GDPR) have set high barriers regarding data handling, which can directly influence discovery data pricing.
- Risk of Penalties: If businesses fail to comply with these regulations, they face severe financial penalties, which can significantly impact their bottom line.
- Cost of Compliance: Adhering to compliance standards can inflate operational costs, which, in turn, may lead to higher prices for end-users.
Furthermore, the ethical considerations surrounding data privacy concern many consumers, who are becoming increasingly selective about the data they share. This growing wariness might prompt a demand for transparent pricing strategies that respect personal information, potentially complicating the pricing models employed.
"In a world where trust is the new currency, securing that trust can be just as expensive as data itself."
Transparency in Pricing Models
Transparency in pricing models is another monumental challenge within the realm of discovery data pricing. A lack of clarity can lead to frustration and skepticism among consumers, which might hinder their willingness to engage with data products or services. In an age where informed decision-making is paramount, your customer base often leans towards providers who are open about their pricing methodologies.
Here are some considerations regarding transparency:
- Breakdown of Costs: Many customers appreciate when they can see a clear breakdown of how prices are determined. This information not only fosters trust but allows for better budgeting decisions.
- Avoiding Hidden Fees: Hidden costs can quickly sour the relationship between businesses and their clientele. Offering straightforward pricing models reduces surprises in invoices, contributing to long-term business loyalty.
However, achieving transparency can lead to friction in competitive markets. Competitors may attempt to exploit transparent pricing as leverage, negotiating better rates or opting for alternative strategies to entice customers. Therefore, striking a balance between revealing sufficient details to satisfy customer needs while maintaining a competitive edge is an art form that companies must master.
In summary, the challenges present in discovery data pricing — particularly in terms of data privacy, security, and pricing transparency — are multi-dimensional. Addressing these challenges is essential for fostering trust and ensuring that businesses can sustainably navigate the complex waters of data pricing.
Future Trends in Data Pricing
Understanding the future trends in data pricing is crucial for anyone looking to navigate the evolving landscape of discovery data. The world of data is constantly shifting, and as new technologies arise and consumer preferences evolve, the implications for pricing strategies change as well. Staying ahead of these trends not only allows organizations to adapt but also positions them to seize emerging opportunities. The intersection of innovative technologies and shifting consumer habits creates a dynamic environment where success hinges on awareness and responsiveness.
Emerging Technologies and Their Impact
Innovative technologies are reshaping the data pricing model in profound ways. For instance, Blockchain technology offers both transparency and security that were previously unmatched in data-sharing agreements. By ensuring that every transaction is recorded and verifiable, it opens a whole new trust dynamic between data providers and consumers. This increase in trust can justify higher pricing models, as customers may be willing to pay more for assurance regarding data integrity.
Moreover, the rise of cloud computing is facilitating real-time access to vast repositories of data. This enables businesses to adjust their pricing models dynamically based on availability and demand. Rather than a static monthly or annual fee, companies can adopt a pay-per-use approach. This flexibility can lead to higher overall consumption and, consequently, increased revenue.
Also, artificial intelligence is playing an indispensable role in refining pricing strategies. AI can analyze historical purchasing data to predict future trends and consumer behavior accurately. This data-driven decision-making means pricing can be tailored to specific customer segments, maximizing perceived value and driving sales. Businesses using AI to refine their pricing methods are often ahead of the curve and better positioned for growth.
"Emerging technologies are not just tools; they are the framework of tomorrow's pricing strategies. Companies must adapt or be left behind."
Evolving Consumer Preferences
Another key aspect of future data pricing is the ongoing evolution of consumer preferences. Today’s customers are more informed and savvy than ever before. They have high expectations regarding transparency and value for their investments in data. Consequently, businesses must carefully consider how they package and price their offerings.
The demand for personalized data experiences is growing. Consumers are increasingly seeking unique, tailored solutions rather than one-size-fits-all packages. This demand creates an opportunity for companies to develop niche pricing strategies focused on customization that can enhance satisfaction and foster loyalty.
Additionally, eco-conscious consumers are becoming more prominent in the marketplace. An increasing number of buyers are drawn to companies that demonstrate sustainable practices in how they handle and price data. Companies that embrace green technologies and show commitment to ethical data sourcing may find themselves with a marketing advantage and the ability to command premium prices.
In summary, the future of data pricing is significantly influenced by both emerging technologies and evolving consumer preferences. To truly excel in this landscape, businesses must remain agile, leveraging technological advancements while keeping a keen eye on the shifting wants and values of their customers. Understanding these facets will not just help in adapting pricing strategies but can also pave the way for strategic innovation that sets a brand apart in an increasingly competitive field.
Culmination and Key Takeaways
In wrapping up our exploration of discovery data pricing, it becomes clear that understanding the nuances of this topic is paramount for organizations looking to enhance their data-driven decisions. Discovery data, characterized by its multifaceted pricing strategies, requires stakeholders to grasp not only the cost structures but also the underlying factors that influence those prices.
One of the crucial elements touched upon throughout the article is that pricing is not merely a reflection of numerical value but also an intricate dance of technology, market demand, and strategic positioning. Companies need to be cognizant of their unique contexts to make informed choices that align with their goals. Failing to do so can lead to financial missteps and missed opportunities.
"In the realm of data, understanding is as valuable as the data itself."
Summarizing Key Points
- Pricing Models Effectiveness: Cost-plus, value-based, and competitive pricing strategies each serve distinct purposes and it's essential for organizations to understand which model aligns best with their operational framework.
- Influx of Technology: Automation and AI have started to reshape pricing models, making it crucial to stay updated with technological advancements.
- Market Conditions: The interplay between supply and demand directly influences data pricing; awareness of market shifts can be a determining factor in strategic pricing actions.
- Regulatory Impacts: With ever-changing regulations governing data usage and privacy, organizations must proactively adapt their pricing structures to remain compliant and ethical.
Final Thoughts on Navigating Data Pricing
Navigating the landscape of discovery data pricing is akin to steering a ship through choppy waters. Without adequate understanding of the pricing models and market conditions, businesses may find themselves adrift.
As we look to the future, organizations should take a proactive stance in monitoring trends that affect discovery data pricing. This includes not only the emergence of new technologies but also changing consumer preferences regarding data usage. By cultivating an adaptive approach, organizations foster resilience in their data strategies, ensuring sustained competitiveness in a progressively data-driven marketplace.
Ultimately, the goal is to develop robust frameworks for data pricing that are transparent, fair, and aligned with business objectives. Successfully mastering this aspect can lead to improved customer relations, optimized resource allocation, and stronger financial performance.