Mastering Business Master Data Management Strategies
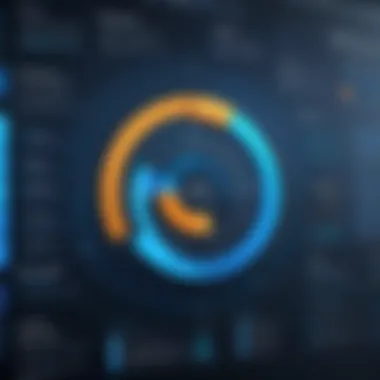
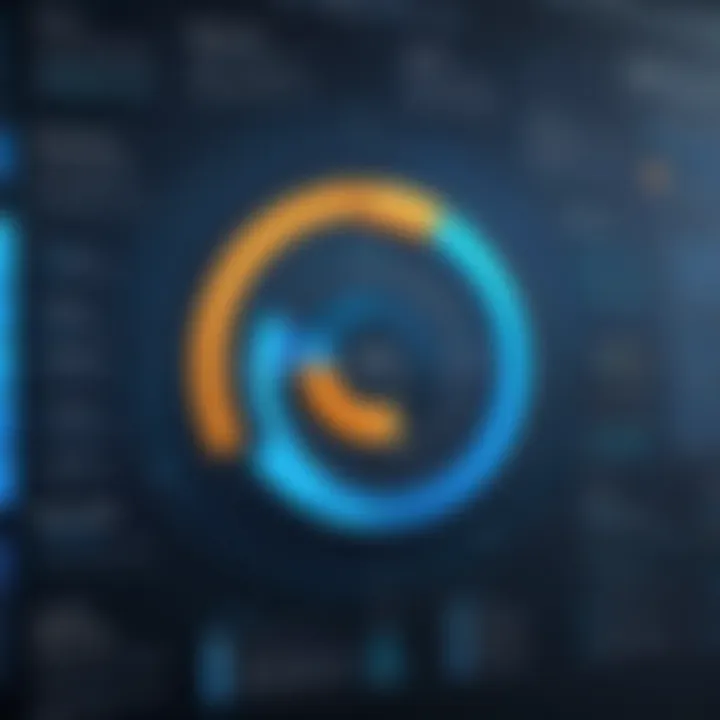
Intro
In today's colossal business ecosystem, organizations grapple with an endless stream of data. From inventory numbers to customer information, the ability to capture and manage this data effectively is not just a luxury; it is a necessity. At the heart of this challenge lies Business Master Data Management (MDM). MDM serves as a beacon guiding companies in their quest for accuracy and consistency among their critical data assets.
Understanding MDM is pivotal for IT and software professionals, along with key decision-makers in both small and large businesses. As firms increasingly rely on data-driven decision-making, the importance of a robust MDM framework becomes undeniable. This article will navigate the intricate landscape of MDM, shedding light on its principles, significance, and various strategies that organizations adopt to manage their master data.
In this exploration, we will also delve into the tools that facilitate effective MDM practices, analyze user experiences with different software, and highlight emerging trends shaping the future of data management. By the end, readers will possess a richer, more nuanced understanding of MDM, empowering them to optimally leverage their business data.
Software Overview
In the realm of Business Master Data Management, software solutions are the backbone underpinning successful data governance. These platforms not only streamline data management processes but also ensure compliance with organizational strategies and regulatory frameworks.
Software Features
When choosing an MDM software, various features can significantly affect how well it aligns with an organization’s goals. Key functionalities include:
- Data Integration: The ability to assimilate data from multiple sources, ensuring that all information is centralized and accessible.
- Data Quality Management: Tools for deduplication, validation, and enrichment that enhance the accuracy and reliability of master data.
- Workflow Automation: Automating mundane tasks to reduce manual errors and bolster efficiency.
- Reporting and Analytics: Robust reporting tools that provide dashboards and visual insights for better decision-making.
- Change Management: Facilitates version control and tracks changes to data over time, enhancing accountability.
These features not only promote transparency but also create a structure that supports data governance initiatives across organizations.
Technical Specifications
MDM software varies widely in capabilities, hence understanding the technical specifications can aid in making informed choices. Some important specifications include:
- Deployment Options: Whether the software is cloud-based, on-premises, or hybrid can influence flexibility and scalability.
- Integration Capabilities: Look for RESTful APIs and support for various data formats which are vital for smooth interoperability with existing systems.
- Scalability: The software’s ability to handle increasing amounts of data as the organization grows is critical.
- User Interface: An intuitive UI can facilitate easier adoption among team members, reducing the learning curve associated with new software.
"Investing in the right MDM software will pay off when you enhance your data quality and streamline operations."
Peer Insights
Understanding how other professionals experience MDM software can greatly inform purchasing decisions.
User Experiences
Many users report that comprehensive training and ongoing support are essential in maximizing the benefits of MDM software. Some organizations have seen profound improvement in data consistency and operational efficiency after onboarding the right tool. Others have encountered challenges related to user adoption and data migration.
Pros and Cons
While MDM software provides numerous advantages, it also comes with its fair share of drawbacks. The principal pros and cons include:
- Pros:
- Cons:
- Enhanced data accuracy, leading to better operational decisions.
- Streamlined workflows, saving time and resources.
- Improved compliance with regulations.
- Initial costs and resource allocation for implementation can be significant.
- Complexity in data integration from diverse sources may pose challenges.
As organizations strategize their approach to Master Data Management, taking into account these experiences and insights can pave the way for seamless and effective implementation, maximizing data utility in the long run.
Prolusion to Business Master Data Management
In today’s fast-paced business environment, organizations are inundated with data coming from multiple sources. Effectively managing this information is a critical factor that often determines success or failure. Business Master Data Management (MDM) serves as the backbone of data governance, streamlining the management of essential data assets while ensuring reliability and accuracy throughout the organization.
MDM encompasses a systematic approach to identifying, managing, and maintaining the organization’s core data entities. This focuses on crucial business information like customer data, supplier details, product specifications, and financial records. Good MDM practices yield significant benefits, such as improved data quality, enhanced operational efficiency, and enhanced decision-making capabilities. In this section, we will delve deeper into the core concepts driving MDM and why it is indispensable in contemporary business practices.
Defining Master Data
Master data refers to the critical business information that is essential for an organization’s operations and reporting. It is typically consistent across various applications and systems and acts as the foundational information that multiple processes depend on. Think of it as the glue holding together different components of a business – without it, organizations can easily fall into chaos and disarray.
Examples of master data include:
- Customer Information: Names, addresses, contact information, and purchase history.
- Product Data: Specifications, categories, pricing, and inventory levels.
- Supplier Details: Company names, addresses, and contact persons.
A well-maintained master data set can provide organizations with a single source of truth, reducing discrepancies and errors. If each department has its own interpretation of customer data, for instance, it can lead to confusion and poor customer service. Therefore, establishing a unified definition of master data and creating logical relationships among data sets is vital.
Key Components of
At the heart of MDM lies a collection of critical components that work in tandem to ensure effective data management. These elements range from data governance to technology platforms, all geared towards safeguarding data integrity and consistency across the business. The main components include:
- Data Governance: Establishing policies and standards for managing master data. This is about setting the ground rules, like making sure the right folks have rights access to the right data.
- Data Quality Management: Continuous monitoring and cleansing of data that allow organizations to retain accurate and up-to-date information.
- Data Integration: Techniques and tools enabling data consolidation from various sources into a cohesive view. Think of integrating information from CRM systems and supply chain databases to present a complete picture.
- Data Modeling: Creating frameworks for how data elements relate to one another, which simplifies how they are used within and across different applications.
- Metadata Management: Keeping track of data definitions, origins, and structures to maintain clarity and usability.
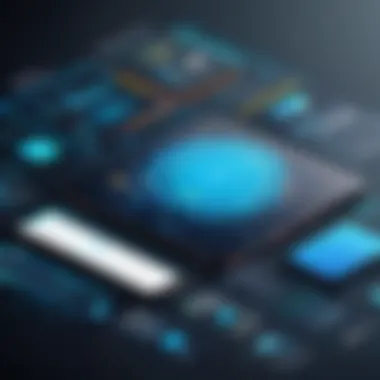
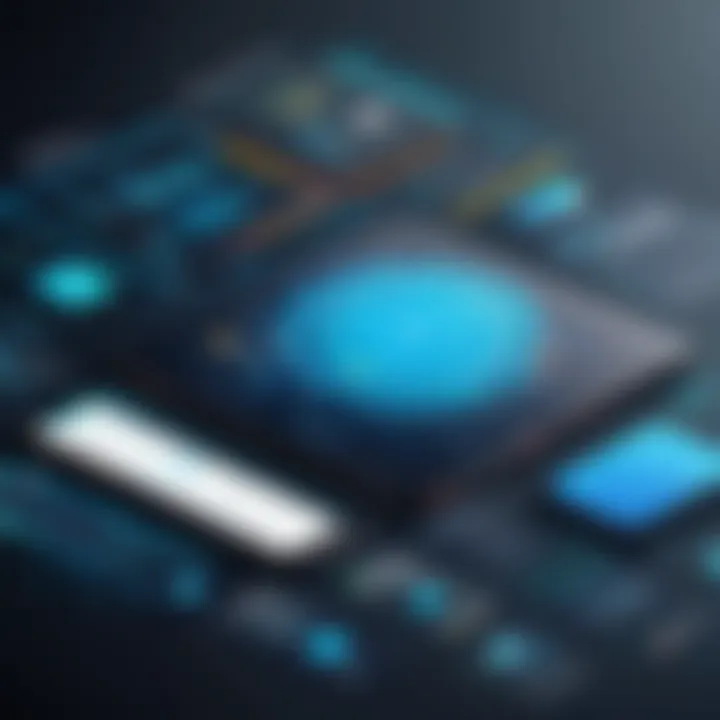
The combination of these key components fosters a robust MDM strategy and propels organizations into data-driven success.
"Master data is not just a byproduct of other processes; it’s the essence of operational success in any organization."
The Importance of in Business Operations
In today’s fast-paced digital world, managing data effectively is no longer the luxury of a few advanced companies; it’s a necessity. Business Master Data Management (MDM) serves as a backbone for ensuring that organizations have access to accurate, consistent, and reliable information at all times. Without a solid MDM strategy, organizations run the risk of operating in silos where crucial data is scattered, leading to errors, miscommunication, and ultimately, poor decision-making. Addressing these challenges signifies the pivotal role MDM plays in enhancing business operations.
Data Accuracy and Consistency
The crux of successful operations hinges on data accuracy and consistency. When data is accurate, decision-makers can trust it to guide their strategies. For instance, consider a retail company that relies on sales data to forecast inventory needs. If the data reflects inaccuracies, the business might overstock certain items while running short on others, leading to lost sales and wasted resources. Hence, investing in MDM ensures that all departments are working with the same set of accurate data.
Furthermore, consistency across data sources minimizes confusion. When everyone in an organization can interpret the same data consistently, collaboration improves. For example, if marketing and sales departments operate with differing definitions of a "lead," it can result in misalignment and inefficiencies. MDM helps establish a singular source of truth, which promotes clarity within teams, driving them all towards shared goals.
"Data is like oil; it's valuable only when refined. Master data management refines the noise into actionable insights."
Enhanced Decision-Making Capabilities
Decision-making can be compared to navigating through a dense fog. Without clear visibility, any course taken is a gamble. MDM serves as the lighthouse, illuminating the path ahead. High-quality data informs better decisions, leading to more successful outcomes.
For instance, consider a financial services firm using MDM to enhance its risk assessment capabilities. By consolidating data from multiple sources—clients, regulatory bodies, and market trends—the firm can build a comprehensive view of the risks they face. This data integration allows for more nuanced strategies, ensuring that decisions are not just reactive but proactive.
Moreover, timely access to clean data enables companies to respond swiftly to market changes. For small and medium-sized enterprises, this agility can spell the difference between capitalizing on an opportunity and missing the boat entirely. A well-articulated MDM approach arms businesses with the right data at the right time, empowering them to make informed choices that align with their strategic objectives.
In summary, the importance of MDM in business operations cannot be overstated. It enhances data accuracy and consistency while elevating decision-making capabilities. As the landscape of business continues to evolve, organizations that prioritize MDM are likely to stay ahead of the game in a competitive marketplace.
Core Principles of Master Data Management
Master Data Management (MDM) serves as the backbone for data strategy within organizations. Understanding and implementing its core principles is essential for ensuring consistency and integrity in a business's data assets. Without these guiding concepts, businesses can easily find themselves in murky waters, grappling with chaos and inefficiency. This section discusses the significance of these core principles and highlights the critical components that can drive successful MDM initiatives.
Data Governance Framework
A solid data governance framework is the cornerstone of effective Master Data Management. This framework outlines how data is managed, ensuring that policies and standards are established to promote accountability and clarity.
- Clear Policies: It's essential to draft clear policies regarding data access and usage. This should cover who can modify data, who maintains it, and what processes there are for data updates.
- Roles and Responsibilities: Establishing a clear chart of roles is vital. Data owners, data stewards, and data consumers need to understand their responsibilities and how they contribute to the overall data management strategy. In this way, everyone knows what’s expected of them, thus reducing misunderstandings.
- Regular Reviews: A governance framework should not be a static document gathering dust on a shelf. Regular reviews and updates based on the latest industry trends or organizational changes are critical to keep the data governance relevant and effective. Organizations can lose the plot if data governance gets sidelined.
Implementing a robust data governance framework ultimately leads to enhanced data quality and compliance with internal and external regulations. This helps in maintaining trust among stakeholders and fortifying the organization against data breaches.
"A strong data governance framework is not just a set of rules, but a culture of responsibility and care around data.'"
Data Stewardship Roles
Understanding data stewardship roles brings clarity and accountability within the MDM processes. Data stewards act as champions for data quality and integrity, and their responsibilities are multifaceted.
- Data Quality Monitoring: Data stewards continuously assess the quality of data being integrated into the system. If issues arise, they ensure that corrective actions are taken. This ongoing monitoring is vital to keep data reliable and pertinent.
- Training and Communication: A data steward often takes the initiative to train other employees on data management best practices. They should be proactive in communicating the significance of maintaining high-quality data and how it affects overall business success.
- Collaboration Across Departments: Data does not exist in a vacuum. Data stewards need to collaborate with various departments to understand their data needs and challenges. This cross-functional collaboration can unveil hidden issues that might compromise data quality and facilitate solutions that benefit the entire organization.
Effectively managing data stewardship roles aids in aligning the company’s data strategy with its broader business objectives. As stewards take ownership, they cultivate a sense of accountability that promotes a disciplined approach to data management.
Methodologies for Implementing
In the quest to harness the power of accurate and reliable data, businesses often hit the crossroads of methodology. The manner in which Master Data Management (MDM) is implemented can set the tone for its effectiveness and longevity within an organization. Choosing the right methodology not only influences the speed of deployment but also determines how well the organization can adapt to changes in data requirements and technology.
Traditional vs. Agile Approaches
When it comes to implementing MDM, two prominent methodologies stand out: Traditonal and Agile approaches. Each approach carries its own flavor and benefits that cater to varying business needs.
With the traditional strategy, organizations often invest considerable time in comprehensive planning. This approach is meticulous, leaning towards detailed documentation and lengthy approval cycles. Many businesses might find reassurance in this method because it emphasizes structure and predictability. For example, a retail company may deploy a traditional MDM system that involves thorough data analysis over several months before even thinking about execution.
On the flip side, the agile methodology strikes a more dynamic chord. The emphasis here is all about flexibility and responsiveness to change. Through iterative development, companies can release smaller chunks of functionality, gather user feedback, and adjust accordingly. Think about a tech startup where the focus is shifting rapidly; they might utilize agile MDM to adapt to new customer insights without getting bogged down by back-and-forth approvals. The quicker feedback loops allow the company to refine their master data management practices in real-time.
Ultimately, choosing between traditional and agile methodologies hinges on key factors such as company size, existing data structures, and future goals. Some organizations even adopt a hybrid model, blending elements from both approaches to effectively navigate their unique landscapes.
Phased Implementation Strategies
A phased implementation strategy serves as a roadmap, guiding organizations through the complex terrain of MDM. This approach tackles the daunting task of deploying MDM in digestible segments rather than attempting a colossal overhaul all at once.
- Assessment Phase: Begin with understanding the current data environment, identifying weaknesses, and establishing a baseline. This isn't just a checklist, it's about cultivating an awareness of where the organization truly stands regarding data quality and usage.
- Planning Phase: After the assessment, businesses need a solid plan. This includes deciding which data domains to tackle first. For instance, a manufacturing firm may choose to focus first on product data integrity before moving on to customer data. It’s about setting priorities based on immediate needs and strategic goals.
- Implementation Phase: This is where the rubber meets the road. Within this segment, organizations should focus on executing the MDM solution in stages, with constant monitoring for any hiccups. If a company starts with product data but encounters issues, they can fine-tune that before rolling out customer data management.
- Review and Optimize Phase: Once an element is live, it’s vital to get feedback and optimize. Continuous evaluation ensures that the data solution evolves alongside the organization’s needs.
By segmenting the implementation in this way, companies can prevent overwhelm, minimize risk, and allow for gradual adjustment. As a result, the entire Master Data Management initiative stands a better chance of being successful while ensuring that the organization realizes its full potential in data stewardship.
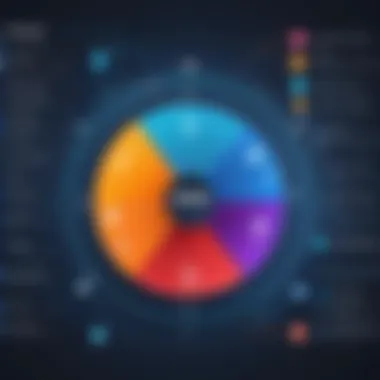
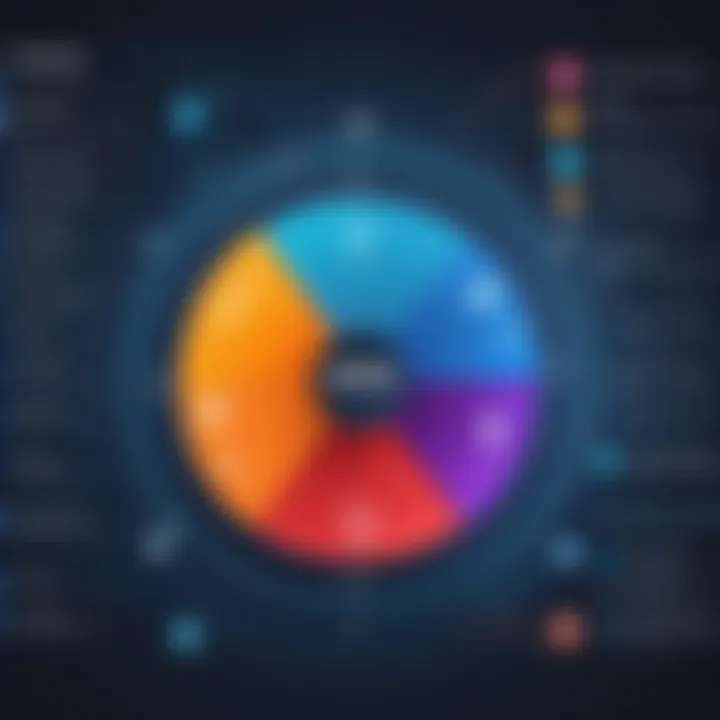
"Implementation is not merely a task; it's a series of thoughtful actions guided by clear strategies."
Navigating the myriad methods of implementation requires careful consideration of company goals and internal dynamics. By understanding the nuances between methodologies and adopting a phased approach, organizations can better position themselves for success in their MDM efforts.
Challenges in Managing Master Data
When diving into the world of Business Master Data Management, one quickly realizes it’s not all smooth sailing. Managing master data comes with its own set of challenges that can throw a wrench in even the best-laid plans. Understanding these hurdles is crucial for anyone looking to implement or improve their MDM strategies. Addressing these issues not only enhances operational efficiency but also fortifies companies against potential data mishaps.
The complexity of managing master data can stem from several sources, notably data silos and fragmentation, as well as regulatory compliance issues. Each of these areas presents unique challenges that require swift and effective responses. The following sections will unpack the significance of these obstacles and offer insight into ways to tackle them.
Data Silos and Fragmentation
Data silos are often the villain in the narrative of effective data management. When data is scattered across different departments or systems, it creates a fragmented view of the business landscape. This fragmentation can lead to inconsistencies in data quality and accuracy, which, as anyone in the field knows, can have cascading effects. A marketing team might be using outdated customer information, while sales could be unaware of recent changes in product pricing. This disconnect can cost time and money, not to mention the possible damage to customer relationships.
- The Effects of Silos:
- Inconsistent Data: Variations in data entries across departments cause confusion.
- Impaired Decision-Making: Leadership struggles when making decisions based on unreliable information.
- Increased Operational Costs: More resources are spent on reconciling discrepancies than on value-generating activities.
As organizations grow, the data silos invariably multiply if proactive measures are not taken. Aiming for an integrated data ecosystem where all departments can access a singular source of truth is essential. This encourages collaboration and ensures everyone is on the same page.
Regulatory Compliance Issues
With an ever-changing regulatory landscape, compliance is not just a ‘nice-to-have’—it’s a must. Companies face scrutiny from various bodies depending on their industry, making it crucial to keep master data in check. Failing to meet compliance standards can lead to hefty fines and reputational risks. This is particularly true in sectors such as finance and healthcare, where data handling is under constant review.
- Core Compliance Concerns:
- Data Privacy: Regulations like GDPR are designed to protect personal data. Companies must ensure that their master data management practices adhere to these laws to avoid legal fallout.
- Data Retention Policies: Organizations must also maintain data for specific periods, which may vary by compliance requirements. Knowing what data to keep and for how long adds another layer of complexity.
- Auditable Data Trails: Maintaining a clear, accessible record of how master data is created, altered, and removed needs to be part of the MDM strategy.
Best Practices for Effective
In the ever-evolving landscape of data management, implementing robust practices is paramount for successful Master Data Management (MDM). Best practices serve as the backbone of effective MDM initiatives, ensuring that organizations can harness the power of their data to drive strategic decision-making and operational efficiency.
Establishing these practices involves a mixture of strategy, governance, and technology. The benefits are extensive and translate into tangible improvements in data accuracy, trustworthiness, and overall business outcomes. When executed properly, these best practices not only alleviate common data-related issues but also foster a culture of accountability concerning data handling throughout the organization.
Establishing Clear Data Governance
Data governance is the compass that guides all MDM efforts. A strong governance framework defines the roles, responsibilities, and processes necessary for maintaining data integrity and security. This thoughtful structure encompasses several key elements:
- Roles and Responsibilities: Clearly defining data ownership helps in reducing confusion and enhances accountability across teams. Data stewards, for instance, have a critical role in ensuring the quality and compliance of master data.
- Policies and Standards: Establishing uniform standards for data formats, definitions, and usage is essential. It prevents discrepancies and confusion that could arise from varied interpretations of data attributes.
- Regular Reviews and Updates: As business needs shift, it's crucial to revisit governance policies regularly to adapt to new regulations, technologies, or business strategies.
Implementing clear data governance ensures that all stakeholders not only understand their obligations but are also engaged in the continuous improvement of data practices. By anchoring MDM in a solid governance framework, businesses can navigate the complexities of data management with far greater ease and effectiveness.
Regular Data Quality Assessments
Data may be perceived as the new oil, but without regular quality checks, it can easily turn into a liability instead of an asset. Conducting ongoing assessments of data quality is essential to ensure that the information used for decision-making is reliable. Here’s how organizations can implement effective data quality assessments:
- Establish Key Performance Indicators (KPIs): Determine clear KPIs to gauge data quality. These might include metrics such as accuracy, consistency, completeness, and timeliness of data.
- Data Profiling: Regular profiling of data allows businesses to get a clear picture of their datasets, identifying anomalies and potential quality issues before they escalate.
- Feedback Loops: Integrating feedback mechanisms where data users can report inconsistencies aids in maintaining the integrity of master data. This can range from automated alerts to user-submitted reports.
- Training and Awareness: Employees who work directly with data need to be trained in data quality standards and practices. Providing this education fosters a greater appreciation for data handling across the organization.
Ultimately, regular assessments can identify potential red flags and help in making informed decisions. By prioritizing data quality, organizations affirm their commitment to excellence, making it a proactive rather than reactive process in the MDM journey.
"Quality is not an act; it is a habit." — Aristotle
Implementing best practices for effective MDM is not a one-off project but an ongoing journey that directly contributes to long-term business success. By focusing on clear governance and regular quality checks, organizations place themselves in a stronger position to harness the full potential of their master data.
Tools and Technologies for
In the realm of Business Master Data Management (MDM), tools and technologies play a pivotal role in shaping how organizations manage their critical data assets. Without the right set of technological solutions, managing master data can become a new-age labyrinth, where inefficiencies and inaccuracies lurk at every turn. Hence, selecting proper tools not only streamlines operations but adds substantial value to business processes, making them pivotal in the grand scheme of MDM strategies.
Software Solutions Overview
The world of MDM is rich with software offerings designed to meet diverse organizational needs. These MDM software solutions come with features that enable businesses to centralize, govern, and manage their master data effectively.
- Central Repository: At the heart of most MDM solutions is a centralized repository. This allows businesses to maintain a single source of truth for their master data, which is critical for ensuring data accuracy and consistency. By funneling all relevant data into a single repository, organizations can reduce redundancy and improve overall data quality.
- Data Integration: Another essential feature of MDM software solutions is data integration capabilities. Integrating data from various sources, including legacy systems, APIs, or cloud applications, ensures that the data landscape remains harmonized. This means increased efficiency, as employees spend less time dealing with data discrepancies and more on generating insights.
Key Considerations for Choosing MDM Software:
- Scalability: The chosen software should grow with the organization. As businesses expand, their data volumes may increase, necessitating flexible solutions.
- User-Friendliness: An intuitive interface encourages wider adoption across teams, which is vital for successful implementation.
- Customization: Every business has unique needs; hence flexible configurations are necessary for optimizing data management processes.
- Budget Constraints: Balancing cost against functionality can be tricky, but investing in robust software is often worthwhile in the long run.
Integrating with Existing Systems
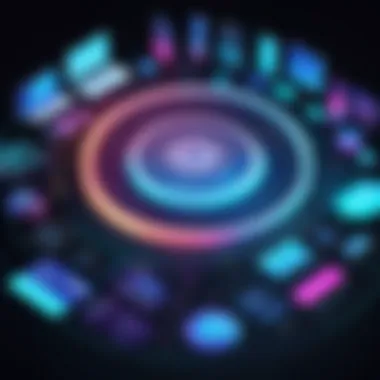
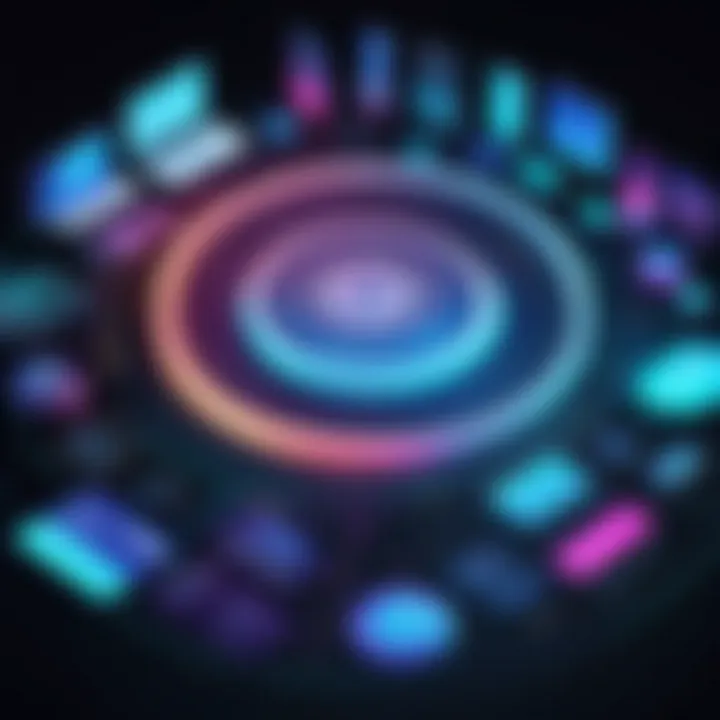
Integrating MDM within existing systems is a task that requires careful planning and execution. After all, businesses often operate with a jumble of applications and platforms, each with its own data management practices. To weave MDM into this fabric, organizations must consider several strategic aspects.
- Understanding Data Flows: It's crucial to map out how data currently flows between systems. By knowing where data originates and where it travels, organizations can identify integration points and minimize disruptions.
- Adopting an Incremental Approach: Instead of overhauling entire systems at once, start with specific datasets or business units. This phased method allows teams to test, learn, and make adjustments before widening the integration scope.
"Success in MDM isn't a sprint; it’s more of a marathon. It takes time to understand and adapt to new paradigms."
- Collaboration Among IT and Business Units: For integration to succeed, both IT and business stakeholders must collaborate closely. Understanding the business context aids IT professionals in designing technical solutions that align with organizational goals.
Ultimately, integrating MDM with existing systems is not merely about technology; it's also about fostering a mindset shift within an organization to embrace data as a strategic asset. This approach can lead to improved data quality, compliance, and, importantly, enhanced decision-making across the board.
The Role of Artificial Intelligence in
Artificial Intelligence (AI) is reshaping the contours of Business Master Data Management (MDM). Incorporating AI technologies into MDM not only boosts operational efficiency but also elevates data governance. Utilizing AI can lead organizations down a road where manual checks become a relic of the past. Instead, AI takes the wheel, allowing businesses to manage their data landscape with precision and agility. From predictive analytics to natural language processing, the infusion of these intelligent systems offers myriad benefits that cannot be overlooked.
Automating Data Quality Checks
Maintaining clean and accurate data can often feel like trying to herd cats. Companies frequently struggle with inconsistencies that slip through when relying solely on manual data management processes. Here, automating data quality checks comes into play.
By employing AI algorithms, businesses can establish a routine for data validation that runs continuously. This automation is akin to having a vigilant sentry keeping an eye on data inputs. Tools can scrutinize new data entries against existing datasets, flagging discrepancies or anomalies that may indicate poor data quality. Automated checks also facilitate significantly faster responses to data issues, enhancing the overall health of the data ecosystem.
Benefits of automating data quality checks include:
- Efficiency: Rapidly identify and rectify issues without the need for constant human oversight.
- Scalability: Handle massive datasets without additional human resources, making it easier to grow operations.
- Consistency: Apply uniform checks across all new and existing data entries.
- Enhanced Reporting: Provide better insights into data quality trends over time.
This level of scrutiny is especially beneficial in industries where data integrity is mission-critical, such as finance or healthcare. By utilizing machine learning, businesses can not only automate checks but also improve them over time as the system learns from past inaccuracies.
Enhancing Data Profiling Techniques
When it comes to understanding data, profiling is crucial. Think of data profiling as being similar to an artist sketching before they paint. Accurate profiles allow for informed decisions about data usage and governance strategies. AI plays an instrumental role in enhancing these profiling techniques.
AI algorithms can analyze large datasets in real-time, identifying patterns and structures that a human analyst might miss. This includes:
- Attribute Analysis: Understand data attributes such as completeness, uniqueness, and constraints at scale.
- Data Relationship Mapping: Automatically identify relationships between disparate data elements, allowing for deeper analyses that were previously labor-intensive.
- Anomaly Detection: Quickly pinpoint outliers or unusual patterns that could signify data quality issues.
These enhanced profiles provide unparalleled insights, which can drive strategic business decisions. For instance, if an AI profiling system reveals that certain customer data attributes consistently contain errors, the organization can proactively address those specific data-entry processes before they create more widespread issues.
Future Trends in Master Data Management
As we peer into the horizon of Master Data Management (MDM), it becomes clear that dynamism will define the terrain. Today's businesses grapple with an ever-increasing pace of change driven by technology, consumer behaviors, and compliance pressures. Understanding the upcoming trends in this field is imperative for organizations aspiring to maintain a competitive edge. Master data must not only be accurate and consistent—it must adapt and evolve continuously.
Real-Time Data Management Solutions
The surge in real-time data management solutions symbolizes a paradigm shift for MDM. Gone are the days when organizations could afford to process data in batches and hope for the best. The advent of technologies like cloud computing and advanced analytics allows businesses to access and analyze data instantaneously. This change has profound implications.
- Increased Agility: Businesses can make swift decisions based on current data rather than relying on outdated snapshots.
- Enhanced Customer Experiences: Real-time data enables more personalized user experiences, making organizations more responsive to customer needs.
- Proactive Decision Making: Companies can act on trends as they emerge, allowing for timely interventions.
With the rise of Internet of Things (IoT) devices, data streams flow in constantly, and managing this inflow effectively is more important than ever. Companies like Snowflake and Talend exemplify how embracing such technologies can turn data into actionable business intelligence, reducing operational bottlenecks.
Evolving Compliance Requirements
In the era of stringent regulations, evolving compliance requirements will inevitably shape Master Data Management. Governments across the globe continually push for greater transparency and accountability, prompting organizations to take a hard look at their data policies.
"In a world of rapid change, the only constant is the requirement for compliance – adapt or risk severe repercussions."
This brings forth several considerations for businesses:
- Changing Regulations: Compliance isn't static; businesses must stay abreast of changes, whether it's data protection laws like GDPR or industry-specific regulations.
- Integration with Risk Management: Companies need to embed compliance monitoring into their MDM systems to quickly assess and mitigate risks.
- Investment in Technology: Advanced tools that automate compliance checks will become increasingly valuable in light of more rigorous audits and inspections.
Ending
In this article, we've traversed the intricate landscape of Business Master Data Management (MDM), shedding light on its many facets that underline its relevance in today's data-driven business environment. The conclusion is paramount as it ties together the insights we've gained, emphasizing key elements that revolve around data accuracy, consistency, and governance.
Summary of Key Points
To encapsulate, here are the main takeaways from our exploration:
- Master Data Defined: MDM is not just about having data—it's about having the right data that is reliable and synchronized across various business units.
- Data Governance Importance: Establishing a solid framework for data governance ensures that data is managed effectively, reducing the risk of inaccuracies and discrepancies.
- Technological Integration: The right tools can significantly improve MDM processes, enabling businesses to address data silos and fragmentation.
- AI and Automation's Role: Artificial intelligence emerges as a linchpin in automating data quality checks, enhancing profiling techniques, and facilitating real-time data management strategies.
- Future Trends: Acknowledging evolving compliance requirements and adopting real-time management solutions paves the way for adaptability in MDM practices.
These points intertwine to form a robust body of knowledge aimed at empowering IT professionals, software developers, and business leaders to navigate the complexities of managing their master data.
The Importance of Continued Adaptation
In closing, it is essential to recognize that the world of MDM is anything but static. The dynamics of business operations are constantly in flux, influenced by technological advancements, regulatory changes, and shifting market demands. Businesses must, therefore, foster a culture of continuous adaptation within their MDM strategies.
- Embracing Change: Organizations should remain open to updating their practices based on new insights and developments in the MDM landscape. This approach is crucial for not only compliance but also for maintaining competitive advantage.
- Training and Development: Continuous training programs for employees focused on the latest MDM technologies and best practices can bolster data management capabilities. This engagement encourages a proactive rather than reactive stance, ensuring readiness for future challenges.
- Feedback Loops: Establishing feedback mechanisms helps in recognizing where systems may fall short and highlights areas for improvement. Be it through regular assessments or utilizing data analytics insights, this practice can serve as a catalyst for refining MDM efforts.
Ultimately, the essence of effective MDM lies in adapting to the evolving landscape of data management. Organizations that embrace this principle are likely to excel in harnessing the power of their data—making better decisions and driving operational success.