Navigating Big Data Analysis Companies and Their Impact
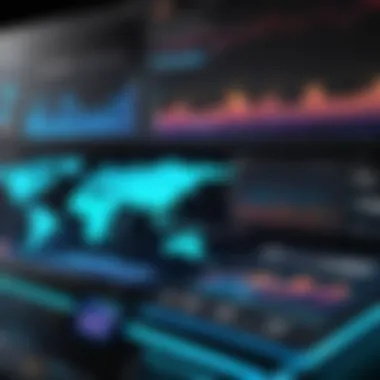
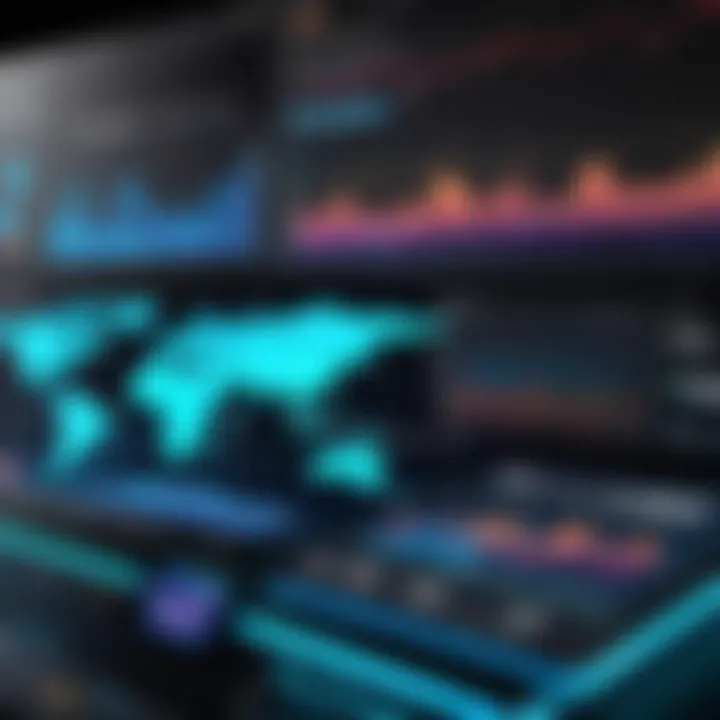
Intro
In today’s fast-paced digital world, the ability to glean meaningful insights from vast amounts of data is not just an advantage; it's a necessity. Whether it’s a small startup navigating uncertain waters or a well-established enterprise looking to fine-tune its operations, the role of big data analysis companies is increasingly prominent. These firms craft intricate frameworks that enable organizations to decode complex datasets and distill them into actionable strategies.
The landscape of big data analysis is a vibrant tapestry woven from various methodologies, innovative tools, and technological advancements. Each company brings its unique flavor to the table, making it imperative for professionals to understand the nuances involved.
Moreover, data-driven decision-making stands as a cornerstone for businesses aiming to stay ahead of the game. From healthcare to finance and marketing to manufacturing, insights derived from data empower companies to evolve, make informed choices, and enhance customer experiences. Understanding the contributions of key players in this field helps clarify how organizations can optimize their operations and achieve growth through strategic data utilization.
As we embark on this exploration of big data analysis companies, we will look closely at their operational strategies, the comprehensive software offered, and insights drawn from users' experiences.
By peering into this complex yet fascinating realm, tech-savvy professionals will gain the knowledge necessary to navigate the sea of data solutions available today, ensuring they are equipped to make the best decisions for their organizational needs.
Prelude to Big Data
In the current business landscape, the significance of big data cannot be overstated. Organizations large and small, across industries, are harnessing vast amounts of information. The way data flows, evolves, and influences decision-making is something every IT professional, software developer, and business leader needs to grasp. Understanding big data is akin to holding the key to a treasure chest filled with insights ready to be unlocked.
Defining Big Data
Big data refers to a colossal volume of structured and unstructured data that inundates a business on a day-to-day basis. It isn't just about the amount of data, though—the velocity at which it's generated, the variety of formats it comes in, and the veracity of its quality all come into play. In plain terms, you can think of it as the sheer mass of information overflow, which traditional data processing applications cannot adequately handle.
The definition often revolves around the three Vs:
- Volume: The sheer amount of data.
- Velocity: The speed of data in and out.
- Variety: The different types and sources of data.
However, more recent discussions have also introduced a fourth V—Veracity—which emphasizes trustworthiness in data.
The Importance of Big Data
Big data holds transformative power for contemporary businesses. It enables a greater understanding of customer behaviors, predictions about future trends, and enhanced operational efficiencies. In an era where organizations compete fiercely, making informed decisions can mean the difference between success and failure; hence, leveraging data is key.
Here are a few benefits of embracing big data:
- Improved Decision Making: With robust analytics, organizations can make more informed strategic choices.
- Enhanced Customer Insights: By analyzing customer interactions data, businesses can tailor their offerings.
- Operational Efficiency: Data-driven insights streamline processes, leading to cost savings.
In the digital age, not utilizing big data effectively is like sailing a ship without a compass.
Additionally, embracing big data opens the doors to innovation. From machine learning algorithms that predict trends to statistical programs that perform deep dives into operational performance, the possibilities are vast.
In summary, as organizations navigate the complexities of modern markets, the grounding principles and practices of big data become indispensable. It’s not just about having data; it’s about understanding and extracting value from it. By properly handling and interpreting big data, companies can elevate themselves to new heights of efficiency and profitability.
Big Data Analysis Companies
Big data analysis companies play a pivotal role in navigating the vast ocean of data that organizations create and collect. By leveraging analytical services, these firms help businesses to transform raw data into actionable insights, which ultimately drives smarter decision-making. The ability of these companies to sift through millions of data points allows organizations to focus on what truly matters - understanding their customers, optimizing operations, and predicting trends.
Overview of the Industry
The landscape of big data analysis is diverse and dynamic, populated by various players ranging from startups specializing in niche markets to established tech giants with comprehensive solutions. The industry encompasses several key sectors, including data storage, processing frameworks, and analytical tools. The ongoing digital transformation across industries amplifies the demand for innovative data solutions.
A few important characteristics of the industry include:
- Scalability: Many big data analysis companies are built to scale operations, accommodating an ever-growing influx of data.
- Real Time Insights: Companies are investing in technologies that facilitate real-time analysis, empowering clients to react promptly to market changes.
- Interdisciplinary Approaches: This field marries various disciplines, including statistics, computer science, and domain-specific expertise, creating hybrid models of service delivery.
A noteworthy trend is the shift toward AI-driven analysis. As algorithms become sophisticated, companies are harnessing machine learning to automate and optimize data workflows.
Key Players in Big Data Analysis
When discussing key players in this arena, several companies emerge as leaders, shaping the direction of the industry. On the radar are:
- IBM: With Watson and other offerings, IBM leads in integrating AI and machine learning into big data solutions. They provide versatile platforms tailored to various industries.
- Amazon Web Services (AWS): AWS has a firm hold over cloud-based data solutions. With powerful tools like Amazon Redshift and AWS Glue, it offers inexpensive and efficient ways to analyze data.
- Cloudera: Well-known for its contributions to open-source software, Cloudera empowers organizations with a comprehensive suite of tools for data management and analysis.
- Palantir Technologies: They focus on government and enterprise data analytics, providing platforms that support complex and large-scale data operations.
"In a world awash with data, the insight you can draw from it can set you apart from the competition."
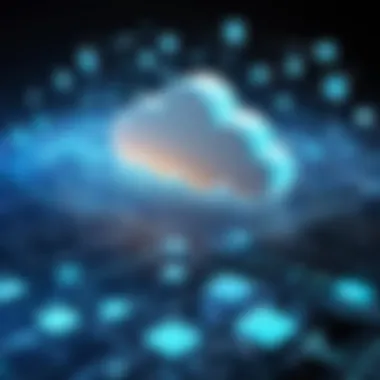
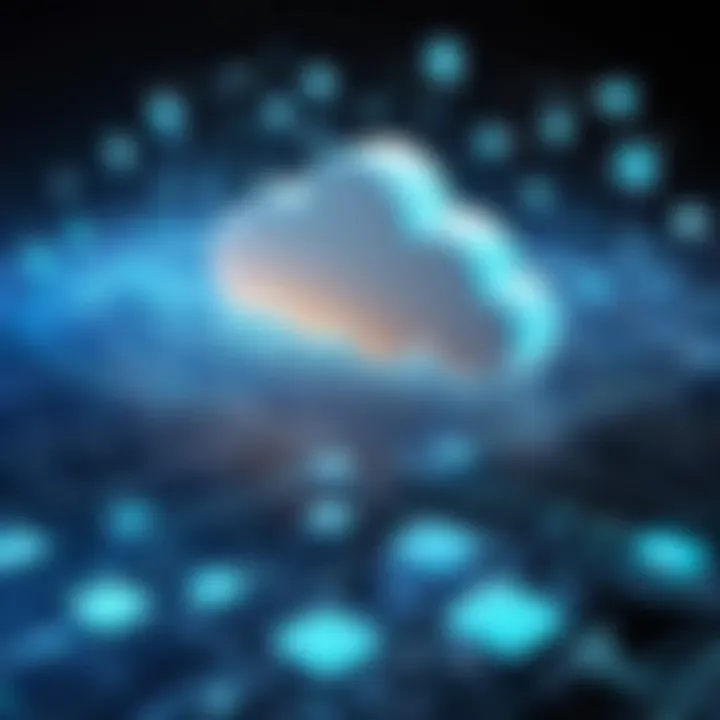
Emerging players are also making their mark. Companies specializing in niche sectors or innovative approaches often present unique solutions that challenge the industry status quo. Startups frequently leverage new technologies to differentiate themselves, focusing on specific industries like healthcare or finance.
Moreover, the collaborative strategies many of these firms employ, such as partnerships or open-source contributions, signify a communal approach to data analysis, fostering a rich ecosystem of shared knowledge and resources.
As this industry matures, keeping an eye on regulatory changes around data privacy and security will be crucial for these organizations. Staying compliant while delivering cutting-edge analytics presents ongoing challenges, yet serves essential for credibility and trust in the eyes of clients.
Technologies Used in Big Data Analysis
Understanding the technologies used in big data analysis is vital for anyone looking to navigate today's data-driven landscape. These technologies shape how data is stored, processed, and turned into valuable insights. It's like laying the groundwork for a well-built structure. Without the right tools, even the most important data can become just a pile of sand without purpose.
Data Storage Solutions
When it comes to big data, storage is king. Companies generate volumes of data at a breakneck pace and choosing the right storage solution is critical. Traditional databases often fall short due to scalability limitations. This is where cloud storage solutions, such as Amazon S3 and Google BigQuery, step in. They offer flexibility and the ability to handle varying data types and sizes, so businesses can scale as needed.
Moreover, NoSQL databases, like MongoDB or Cassandra, are particularly good with unstructured data. This means that whether you're dealing with social media posts, customer interactions, or sensor data, these systems can store it effectively.
"Data is the new oil, but without the right storage, it’s a mess of raw resources."
Data Processing Frameworks
Once data is stored, it must be processed. Data processing frameworks, such as Apache Hadoop and Apache Spark, enable organizations to efficiently analyze vast quantities of data. Hadoop provides a framework for distributed storage and processing, allowing organizations to break down large data sets into manageable pieces. Its flexibility supports different programming languages, which is a significant plus.
On the flip side, Apache Spark offers speed and efficiency. It's like comparing a lumbering truck to a sleek sports car. Spark processes data in-memory, making it significantly faster than traditional systems, especially for tasks requiring real-time analytics.
Analytical Tools and Techniques
Analytical tools are crucial for transforming raw data into understandable insights. Companies today must utilize multiple tools and techniques to cater to diverse analytical needs.
Machine Learning Algorithms
Machine learning algorithms have emerged as a fundamental component of big data analysis. These algorithms learn from data patterns, helping businesses predict trends and make informed decisions. For example, a retail company might use sales data to predict what products will be in high demand. This ability to forecast can lead to significant competitive advantages.
A key characteristic of these algorithms is their capability to continuously improve over time as they are fed more data. It leads to increasingly accurate models. However, a notable disadvantage is the requirement for clean, well-structured data. If the data is messy or incomplete, the algorithms may offer misleading insights, ultimately causing more harm than good.
Statistical Analysis
Statistical analysis methods form the backbone of data-driven decision making. They allow analysts to discern relationships within data. For instance, correlation coefficients can indicate how two variables behave together. This method's strength lies in its ability to derive actionable insights from data distributions and variability.
A unique feature of statistical analysis is its focus on interpreting results meaningfully. However, one drawback is that it often relies on samples rather than whole populations, which can introduce errors if the samples are not representative.
Data Visualization Techniques
In the world of big data, data visualization techniques offer a window into the story behind the numbers. Tools like Tableau and Power BI help convert complex data sets into easy-to-understand visuals. This representation supports decision-making processes significantly. Managers can quickly grasp trends and patterns rather than sifting through endless spreadsheets.
The critical aspect of data visualization is its ability to highlight key insights at a glance. It also encourages collaboration across departments, as everyone can comprehend the visualized data. The downside, however, can be the oversimplification of data, leading to misinterpretation if one does not dive deeper into the context.
In summary, the technologies deployed in big data analysis are essential for unlocking insights that drive business success. From storage solutions to the analytical tools that sift through data, each component plays a pivotal role in transforming raw numbers into strategic decisions.
Business Applications of Big Data Analysis
The role of big data analysis in today's corporate landscape cannot be overstated. Organizations are harnessing vast quantities of data to drive decisions that impact their operational efficiencies and bottom lines. Utilizing Data Analytics effectively can radically change how businesses approach their strategies, ensuring they stay ahead of their competition.
Key Elements of Business Applications
Among the many sectors leveraging big data, we observe significant transformations within healthcare, finance, and retail. These domains exemplify the richness of insights that can be extracted from rigorous data analysis, leading to enhanced services and profitability.
Understanding the specific benefits of these applications empowers stakeholders to make informed decisions about data investment and partnerships.
Healthcare Industry Innovations
In healthcare, big data analysis is paving new paths in patient care, operational efficiency, and preventive measures. Hospitals and clinics are employing advanced analytics to sift through patient records, genetic data, and treatment outcomes. The ability to analyze this information timely caters to personalized medicine, meaning treatments can be tailored to individual patient needs.
One example is the use of predictive analytics for patient admissions, which allows healthcare facilities to allocate resources effectively, reducing wait times and improving patient satisfaction. Beyond operational improvements, big data also supports epidemiological research, identifying patterns in disease spread that can lead to better public health strategies.
Benefits of Big Data in Healthcare:
- Enhanced patient outcomes
- Predictive analytics for resource allocation
- Targeted treatment plans
- Improved operational efficiency
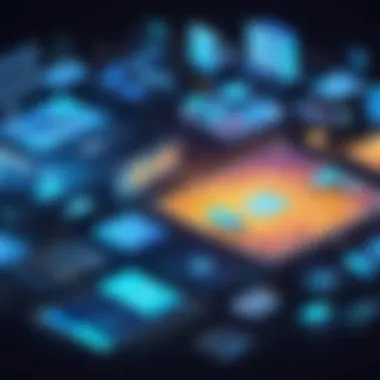
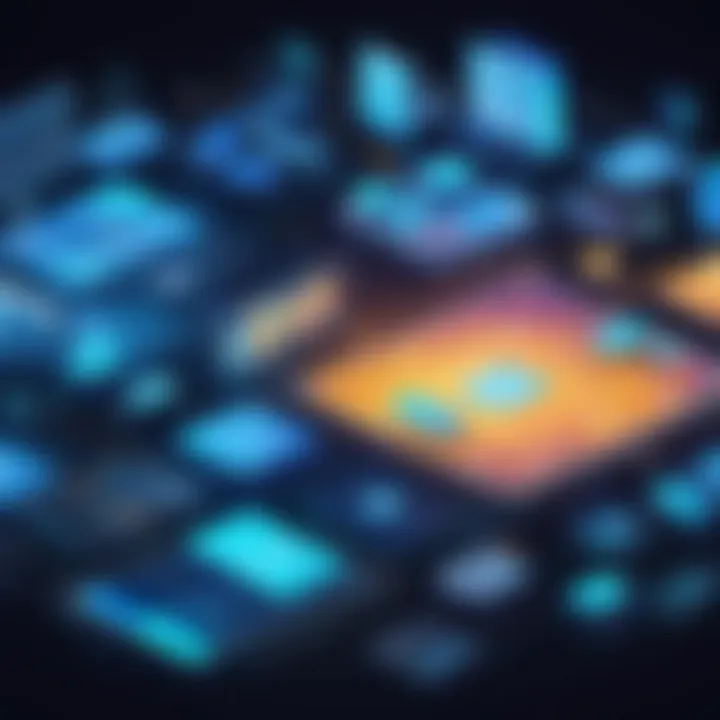
Financial Sector Transformations
The financial services industry has seen dramatic shifts thanks to big data. Companies are employing sophisticated algorithms to detect fraud, assess risks, and enhance customer engagement. With financial transactions generating enormous amounts of data daily, businesses like JPMorgan Chase and Goldman Sachs utilize analytics to fine-tune their offerings and improve client experiences.
Risk management is particularly crucial in finance. By analyzing historical data and current trends, firms can forecast potential market shifts or identify credit risk more accurately. For instance, machine learning models are constantly evolving, allowing institutions to adapt evidence-based strategies, thus ensuring they remain competitive in a fluctuating economy.
Significant Applications in Finance:
- Fraud detection systems
- Risk assessment modeling
- Client personalization through predictive targeting
Retail Insights and Strategies
In the retail space, big data is reshaping how companies understand consumer behavior and optimize their operations. From inventory management to marketing strategies, retailers such as Walmart and Amazon leverage analytics to ensure they meet customer demands efficiently.
These companies can track purchasing trends, analyze customer reviews, and even adjust prices dynamically based on market data. Such strategies lead to improved customer loyalty and increased sales.
"Data is the new oil; it's valuable, but if unrefined it cannot really be used. So researchers and organizations have to find ways to extract actionable insights from this vast pool of information."
In summary, big data analysis forms the backbone of innovation and strategic advancement across multiple sectors. Businesses that embrace these applications are not only improving their operations but are also positioning themselves as leaders in their respective fields, capitalizing on the advantages that data-driven decision-making affords.
Challenges in Big Data Analysis
Dealing with big data isn't just about having the right tools or techniques; it involves navigating a multitude of challenges that can affect the strategy and execution of data analysis projects. These challenges can significantly impact not only the quality of insights derived from data but also the trustworthiness of the findings. Companies venturing into big data analysis must therefore take a step back to assess the multifaceted hurdles that lie ahead. In this section, we will delve into two critical challenges: data privacy concerns and handling data quality issues.
Data Privacy Concerns
In the age of information, data privacy stands as a towering challenge for organizations of all sizes. With regulations like the General Data Protection Regulation (GDPR) and California Consumer Privacy Act (CCPA) making waves, the stakes have never been higher. Companies are often caught in a bind; while they wish to harness the power of data for insights, they must also safeguard individual privacy rights.
Why It Matters:
Infringements of privacy can lead to severe repercussions ranging from hefty fines to irreparable damage to a company’s reputation. A breach not only affects the users but can also result in loss of trust among customers, which is often tough to rebuild. For instance, a 2021 report indicated that 43% of consumers admitted they would stop using a brand if their data was mishandled.
Strategies To Consider:
- Implementing Data Encryption: Encrypt sensitive data, making it less accessible to unauthorized users.
- Comprehensive Data Governance: Establish clear guidelines on data usage, retention, and sharing among employees.
- Transparency Efforts: Clearly communicate to users how their data will be used, keeping them in the loop about privacy policies.
These steps not only assist in compliance with various regulations but might also act as a unique selling point for data privacy-conscious consumers.
Handling Data Quality Issues
Data quality is another crucial aspect that can make or break a big data initiative. Poor data quality can stem from various sources, such as outdated information, human error, or inconsistencies in data entry. These issues can obscure the true story data is trying to tell, leading to misguided business decisions and wasted resources.
Reasons for Concern:
Research shows that businesses lose around 20-35% of revenue due to poor data quality. This statistic is a stark reminder of the importance of ensuring that the information being processed is consistent, accurate, and up-to-date.
Techniques to Improve Data Quality:
- Regular Audits: Conduct regular checks to identify and rectify anomalies in data sets.
- Standardization of Data Entry: Implement consistent coding systems for data entry to minimize human error.
- Utilizing Data Cleansing Tools: Leverage technology to automatically clean and refine data inputs, reducing the amount of manual work involved.
By prioritizing data quality, organizations can significantly enhance the reliability of their analysis, leading to more informed decision-making processes.
Future Trends in Big Data Analysis
The realm of big data analysis is constantly evolving, and staying ahead of the curve is no small feat. As the landscape changes, so do the trends that define the future of this industry. Understanding these trends is crucial not just for companies engaged in data analysis but also for businesses relying on their insights for strategic decision-making. This section dives into the significant trends shaping the future of big data analysis, emphasizing the key advancements and considerations that businesses must keep in mind.
The Rise of Real-time Analytics
Real-time analytics has become a cornerstone of modern data processing. Businesses can no longer afford to operate on outdated information; timely feedback is as good as currency in today’s fast-paced market. Companies can analyze data as it is generated, allowing for immediate insights and actions. This shift is driven primarily by the increased demand for agility in decision-making processes.
Key Advantages:
- Timely Decision Making: Organizations can respond to a changing business environment almost instantaneously.
- Enhanced Customer Experiences: Personalization based on current customer interactions leads to better service.
- Immediate Risk Management: Companies can identify and mitigate issues before they escalate.
The rise of real-time analytics isn't without its challenges. There are hurdles in terms of data integration, scalability, and the need for advanced infrastructure to support such capabilities. Businesses aiming to adopt real-time analytics must weigh the investment against potential boosts in efficiency.
Integration of Artificial Intelligence
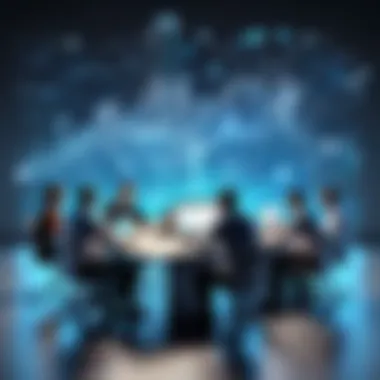
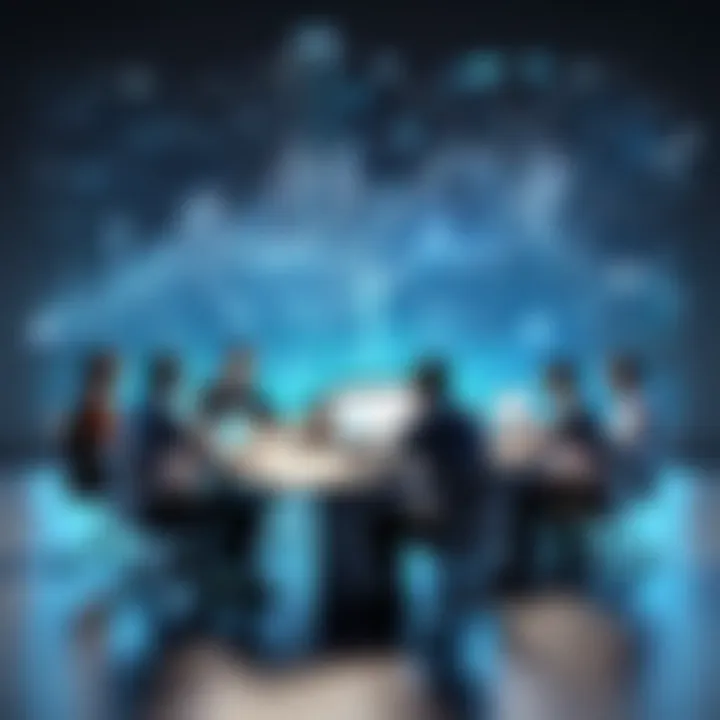
Artificial Intelligence (AI) is not just a buzzword; it's rapidly becoming a vital component of big data analysis. By integrating AI, companies can enhance their analytical capabilities significantly. Machine learning algorithms and predictive analytics are helping firms sift through vast amounts of data to reveal patterns and trends that human analysts may miss.
Reasons for Integration:
- Automation of Processes: AI can automate routine data processing tasks, freeing analysts to focus on strategic initiatives.
- Improved Accuracy in Predictions: Enhanced models lead to more accurate forecasts which are critical for long-term planning.
- Scalable Solutions: AI enables businesses to scale their data processing capabilities efficiently without a proportional increase in resources.
However, reliance on AI also brings about ethical considerations regarding data privacy and ensuring fairness in algorithms. Failing to address these areas can lead to mistrust among consumers and stakeholders.
Expanding Role of Cloud Computing
Cloud computing is revolutionizing how businesses handle data analysis. The flexibility and scalability provided by cloud technologies are creating fertile ground for sophisticated data analytics without the limitations imposed by traditional on-premises systems. And as companies shift to cloud solutions, they're finding cost-efficiencies and enhanced collaborative tools.
Benefits of Cloud Computing in Big Data Analysis:
- Scalability and Flexibility: Businesses can effortlessly scale their operations based on demand.
- Cost-Effectiveness: By using cloud services, companies save costs on infrastructure and maintenance.
- Greater Collaboration and Accessibility: Teams can access data and analytical tools from virtually anywhere, facilitating remote work and global collaboration.
The shift to the cloud isn't just a trend; it’s becoming a necessity for companies looking to leverage big data effectively. However, organizations must also keep an eye on security and compliance measures associated with cloud technologies.
"The future of big data analysis isn’t just in collecting data; it's about making informed decisions swiftly—powered by technology like AI and cloud computing."
In summary, the trends in big data analysis are not just about technologies and processes; they represent a fundamental shift in how organizations interact with data. Businesses must adapt and evolve with these trends to remain competitive in today’s data-driven marketplace.
Selecting a Big Data Analysis Company
Choosing the right big data analysis company is a pivotal step for enterprises looking to harness the power of data. As organizations increasingly rely on data to drive their decisions, it becomes crucial to work with a partner who can deliver valuable insights and tailored solutions. This section delves into the key considerations that should influence this selection process, offering insights for IT professionals, software experts, and business leaders alike.
Assessing Company Capabilities
When evaluating a big data analysis firm, the first thing to do is assess their capabilities. Not all companies are created equal, and their expertise can vary significantly.
- Technical Proficiency: Check if the company has experience in the technologies relevant to your needs. Are they proficient in machine learning, statistical analysis, or data visualization techniques? Familiarity with tools like Apache Hadoop or Spark is essential.
- Industry Experience: It’s beneficial if the firm has worked in your industry. They may understand specific challenges and regulations that can impact data handling and analysis effectively. For example, a company with a solid track record in healthcare may have insights that a generic software vendor might miss.
- Project Portfolio and Case Studies: Look for documented success stories. If the company has helped businesses improve efficiency or enhance decision-making through their analysis, it’s worth noting. Reading about specific projects can give a glimpse into their methodologies and effectiveness.
- Client Testimonials: Customer reviews can provide clarity on the firm's strengths and weaknesses. Sometimes, firsthand experiences are more illuminating than any press release.
- Data Compliance Standards: As privacy regulations tighten, ensuring that the company adheres to data protection laws like GDPR or HIPAA is indispensable. Verify that they implement best practices in data security and compliance.
It's essential to have a comprehensive understanding of these elements before diving into a partnership. Ultimately, a firm’s depth in capabilities can lead to fruitful long-term collaborations.
Understanding Pricing Models
Pricing models can be a complex affair. Understanding them is key to making a well-informed decision that balances quality and cost. Consider the following aspects:
- Pay-per-Use vs. Subscription: Companies may offer different pricing arrangements. Pay-per-use can be ideal for smaller or infrequent projects, while subscriptions might be more economical for ongoing data needs or larger ventures.
- Transparent Pricing Structures: It’s crucial to ask for a clear breakdown of costs, including any hidden fees. Clarity can prevent complications later in the partnership.
- Analytics Packages: Some firms bundle their offerings into packages, which can provide comprehensive solutions at a more attractive price point. This can include tools for data storage, processing, and visualization all in one go.
- Customization Pricing: Understand how customizable the services are and how that impacts pricing. Tailored solutions might come at a premium, but they could also deliver better results for niche needs.
- Freemium Models: Some companies offer basic services for free. While attractive, it’s essential to assess whether the expanded functionalities justify potential future costs.
As organizations navigate these intricate waters, understanding and evaluating pricing models not only aids in budgeting but also ensures candidates for selection aren’t just financially viable but also strategically aligned with business goals.
"Selecting a suitable big data analysis partner is akin to picking a co-pilot. Their expertise can steer you clear of turbulent data storms and toward a runway of insights."
Ultimately, a thoughtful approach to selecting the right big data analysis company is necessary for reaping the maximum benefits of data-driven initiatives.
Ending
In the context of this article, the conclusion serves as a critical component in crystallizing the multifaceted aspects of big data analysis companies. It's not merely a wrapping up of points but rather a reflection on the narrative woven throughout the preceding sections.
Summary of Key Insights
Through this exploration, key insights emerge about the role and significance of big data analysis firms in today's business environment. First and foremost, these companies are integral in translating vast troves of data into actionable insights. The impact spans multiple sectors, such as healthcare, finance, and retail, each leveraging data analytics for enhanced decision-making and operational efficiency.
Furthermore, the technologies they employ—from storage solutions to machine learning algorithms—are essential for processing and extracting value from data. For instance, the use of cloud computing has revolutionized how companies approach data management, allowing them to scale and adapt in real time.
In summary, big data analysis not only drives innovation but also encourages businesses to approach challenges from a data-informed perspective. A quick list of these insights includes:
- The increasing need for real-time data processing.
- The importance of data privacy and quality.
- The growing role of artificial intelligence in analysis.
- The need for businesses to understand the capabilities and pricing models of data analysis services.
The Future of Big Data Analysis
As we peer into the future, several trends become evident. The rise of real-time analytics signifies that companies will need to adapt swiftly to data fluctuations. Integrating artificial intelligence not just in data analysis, but also in decision-making processes, will further redefine how businesses function.
Moreover, the expanding role of cloud computing is likely to continue. Organizations are beginning to understand the flexibility and efficiency that cloud-based systems offer for data storage and processing needs. As these trends unfold, companies that prioritize adapting to new technologies and investing in robust analytical methodologies will likely rise above the competition.
"In the world of big data, your next competitive edge is lurking in the shadows of your data streams, waiting to be illuminated by insightful analysis."
In essence, as businesses continue to harness the power of big data, the insights gleaned will vital in not only surviving but thriving in an ever-changing marketplace.